MARIA ESTEBAN CASAÑAS
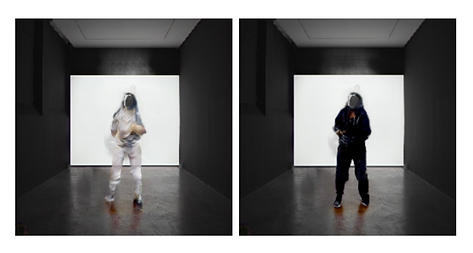
ARTIFICIAL PERCEPTIONS
Massachusetts Institute of Technology
Our world is emulated in Artificial Intelligence, and with it, biases and fictionalities. Through a variety of examples, speculative arguments, and performances, this study explores how biases are produced and fictionalities created through shifting signifiers.
This thesis has a dual voice. It is generated in two versions - one written by me and one developed by a text- producing algorithm I “trained”. As such, and given its generative process, this thesis could be interpreted as a performance even for those who read it.
“Artificial Perceptions” could therefore be understood as rendering a new vision of how Artificial Intelligence can be used to create new content, disclose existing predispositions, and be utilized as a collaborative tool. Shifting signifiers prompts artificial perceptions and allows us to revisit and permutate biases that are intrinsic to AI. It challenges the construction of our understanding of our own “artificial reality” and exposes the cultural idiosyncrasies of the computational discipline.
The term “semiotic deepfakes” is coined as a reaction to excerpts of text generated by the trained model, envisioning how machine learning might mislead the public on authorship. This idea is explored further through the development of Alan Turing’s Imitation Game, allowing the reader to take the role of “interrogator” within this thesis. I use Turing as the foundational premise for the various experiments of my own design in the thesis.
It concludes with a performance between all agents in this thesis, including the committee, the algorithms, and the author, adding to the semiotic discourse in a playful yet unsettling manner.
This Thesis was awarded the 2020 MIT Architecture Thesis Prize.